Green and intelligent: The global economy in the 21st century
Featured technology
Products & Services
Who we’re helping
Everyone
Our role
Boldly accelerating AI for climate action while responsibly managing its environmental impact
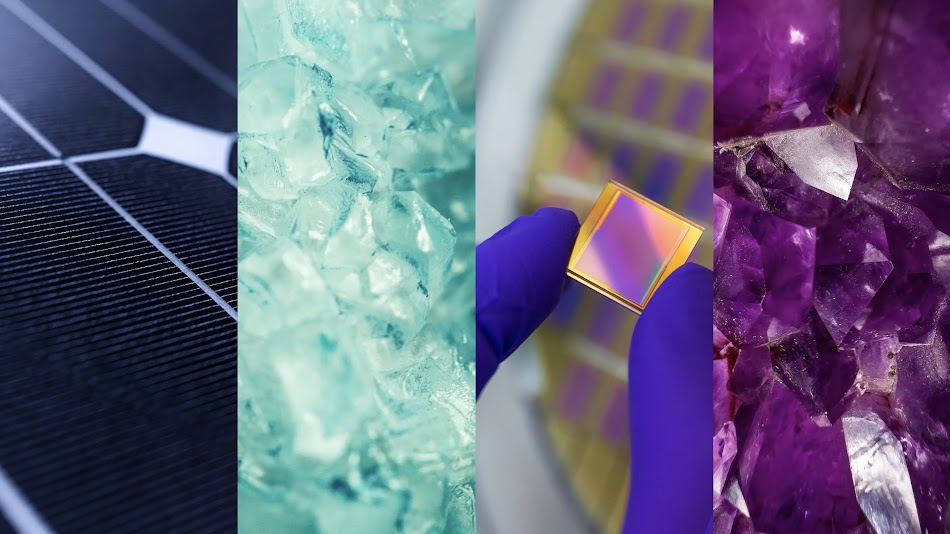
Featured guest post by Nicholas Stern, Chair, Grantham Research Institute on Climate Change and the Environment, London School of Economics
The world has in its hands the potential to apply Artificial Intelligence (AI) and Machine Learning (ML) to drive forward the net-zero transition and give us a chance to stay within 1.5℃. AI and ML can contribute massively to the pace of processes (e.g., process automation, accelerated data processing and forecasting), can drive higher productivity (e.g., energy efficiency and resource productivity), and help to design and run better systems (e.g., how energy, cities, or mobility systems operate as a system and how such systems interact). Together, they can unlock new growth, sustainable, resilient and equitable, whilst managing the immense and urgent risks of climate change, biodiversity loss, and pollution.
Although limited research exists on the extent of the combined growth effect of AI and the low-carbon transition, there is evidence that they compound strongly to accelerate system change. My recent research in collaboration with a range of experts has identified five areas where AI and ML applications could be especially effective in accelerating the low-carbon transition:
1. Improving complex systems: Effective action on climate requires rapid structural transformation in key systems, including cities, land use, transport, industry, and energy. AI can help to redesign such complex systems and run them effectively and efficiently. For example in the energy sector, AI can help to manage predictability in supply-demand balance and improve system productivity. Google DeepMind has demonstrated that such applications can help increase the economic value of wind energy by 20% by reducing reliance on sources of standby power.
2. Encouraging behaviour change: AI and ML technology is already used to identify and predict individual and collective behavioural patterns. This can be applied to help reduce emissions by providing more sustainable choices to consumers that have lower environmental impact. For example, Alipay’s Ant Forest project rewards its users with “green energy points” every time they make a “green choice,” such as using a shared bike to commute to work. In exchange for the points, Alipay plants a tree or protects habitat. AI could help to further advance such mechanisms to encourage behaviours toward sustainable system change given social norms and the actions of others are important drivers of pro-environmental behaviour.
3. Discovering new technologies and solutions: The next 10 years will be crucial to bring clean technologies to market. AI and ML can accelerate the process of scientific discovery in ways which were unimaginable only years ago. For example, more than 2 million theoretical crystal structures were recently identified by Google DeepMind using an AI tool called GNoME—more than 45 times the number of such structures identified so far by science. As shown by a recent study from the London School of Economics, general-purpose technologies are driving the direction of technological change and, in particular, the competition between clean and dirty technologies.
4. Modelling climate systems and policy: Climate change is already having major global impacts, including rising sea levels and increased frequency of extreme weather events. AI and ML can improve understanding the causes of these events, for example by analysing complex interactions between climate change and Arctic sea ice loss. They can also be applied to better design and implement public policies for climate action, with better prediction of their effectiveness in driving choices and behaviours of organisations and individuals.
5. Forecasting impacts to increase adaptation and resilience: One major AI application is in hazard forecasting and improving climate disaster alert systems. Researchers at the British Antarctic Survey and the Alan Turing Institute have developed IceNet, an AI predictive tool that forecasts sea ice levels using data from satellite sensors in climate modelling. Google’s Flood Hub uses AI to help provide more accurate information on riverine floods up to seven days in advance, which forecasts floods in more than 80 countries.
The potential is huge. Yet there are a number of issues, risks, and unknowns that require further thought and attention. Some questions on this topic include:
1. How can we manage the bias inherent in current AI systems—especially biases due to foundational data that’s primarily based on information from the Global North and dominated by English-language knowledge?
2. AI and ML technologies base their future predictions on patterns of past data. We therefore must be careful when using them as guides through structural changes that are likely to be profoundly different from the past. Will AI and ML predictions avoid the traps that have previously led us to systematically underestimating the power of innovation and adoption of low-carbon technologies at scale?
3. As the economy shifts toward a net-zero future and AI and ML applications begin to scale, how can we address concerns around automatization and jobs, fostering a just transition in the face of these two compounding transformations?